Are Data Quality and ALCOA attributes equivalent?
Introduction
The attributes1 utilized to evaluate the integrity of data and records associated with activities covered by the medicines’ manufacturing practices applicable regulation in the life sciences sector is called ALCOA. It is relevant in other areas, particularly pharmaceutical research, clinical, testing, and the supply chain.
Stan Woollen introduced ALCOA2 as a criterion for United States (US) Food and Drug Administration (FDA) Good Laboratory Practices (GLP) inspectors to assess data quality.
In addition to the US FDA, all other worldwide regulatory agencies or competent authorities associate ALCOA with the attributes to assess the quality of data collected to demonstrate the safety and efficacy of medicinal products.
This article compares the attributes of ALCOA in the context of medicinal products authorities and data quality in the context of data engineering to find if the ALCOA attributes can be used to assess data quality.
The following are brief explanations of each component of ALCOA, ALCOA+. ALCOA++, reliability, and Data Quality3 are used in this article. As ALCOA, these contain criteria to assess data collected during the execution of activities covered by practices regulation.
ALCOA
ALCOA is an acronym for Attributable, Legible, Contemporaneous, Original, and Accurate. Each of the criteria is used to assess data quality.
Here's a brief explanation of each component of the ALCOA acronym:
- Attributable
All data should be linked to a specific source or individual related to activities, including data generation, modification or deletion. This link ensures accountability and transparency in the documentation process. - Legible
All records should be clear and easy to read to minimize the risk of misinterpretation or errors (e.g., safeguarding electronic records to ensure data recovery in an emergency). - Contemporaneous
Documentation should be recorded in real-time or as close to the time of the activity as possible. - Original
Original data includes the data and information initially collected and all subsequent data required to fully reconstruct the activity's implementation, including electronic data in automated manufacturing systems (e.g., SCADA, Historian, DCS, and so on). - Accurate
Data accuracy is the degree to which data reflects the valid values or facts it represents4. One example is the records generated during the validation of computer systems that generate, administer, distribute or archive e-record.
Data architecture is critical for organizations because it helps ensure data accuracy.
Adhering to the ALCOA principles helps to prevent errors or misconduct in the documentation process, ultimately contributing to the safety and efficacy of products and services.
The above ALCOA principles have since been updated to ALCOA+.
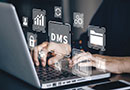
Recommendation
Heidelberg, Germany1-3 April 2025
Good Documentation Practice and Data Integrity
ALCOA+
ALCOA+ expands upon the original ALCOA acronym by adding three additional components to ensure data integrity in regulated industries. The original principles remain with three additions: Complete, Consistent, and Enduring.
ALCOA+ includes:
- Complete
Complete data refers to data that contains all the necessary information required to make accurate and reliable conclusions. It means that all the relevant variables, observations, and measurements have been recorded, and there are all values and data points, including all relevant information necessary to understand the context of the activity. - Consistent
Documentation practices should be standardized across all records, systems, and personnel to ensure uniformity and consistency in data collection. - Enduring
Data should be preserved and maintained over its entire life cycle, including all raw data, metadata, and associated documentation. Enduring is achieved by implementing processes to ensure the data is stored, archived or disposed of safely and securely during and after the decommissioning of the computer system.
Adhering to ALCOA+ principles ensures that data is accurate, reliable, complete, consistent, and well-preserved, which is critical for ensuring regulatory compliance and maintaining data reliability throughout the data life cycle.
ALCOA++
ALCOA++ is an extension of the ALCOA and ALCOA+ components, including additional requirements to ensure data integrity in highly regulated industries.
ALCOA++ includes:
- Traceable
All data should be traceable to its raw data, with a clear audit trail that demonstrates the history of the data from its creation to its current state. - Available
Data should be readily available and accessible to authorized personnel as needed while ensuring appropriate security controls are in place to prevent unauthorized access or modification. Data architecture is critical for organizations because it helps ensure data is available when needed. - Secure
Data should be protected against unauthorized access, modification, or destruction, and security controls should be in place to ensure data confidentiality, integrity, and availability.
Adhering to ALCOA++ principles ensures that data is accurate, complete, consistent, and well-preserved but also traceable, available, and secure, which is crucial for ensuring regulatory compliance, maintaining data quality, and protecting sensitive information.
By implementing these principles, organizations can maintain the highest data reliability and trustworthiness standards, which are critical in highly regulated industries such as healthcare, pharmaceuticals, and finance.
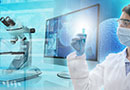
Recommendation
6/7 May 2025
Audit Trail Review for Computerised Systems in Analytical Laboratories - Live Online Training
Data Quality attributes5
Data quality is healthy at any stage in its lifecycle6 and is the foundation for deriving valuable insights and driving better decision-making processes.
Data quality is determined by several attributes, including accurate, auditable, conform, complete, consistent, with integrity, validity, reliable, timely, and consistent with the intended use. These attributes ensure that the data is both correct and valuable.
- Accurate.
All data should be precise, complete, and truthful, reflecting what occurred. Data architecture is critical for organizations because it helps ensure data accuracy.
Data accuracy is the degree to which data reflects the valid values or facts it represents.
- Auditability
Data auditability refers to the ability to trace and verify the raw data, integrity, and lineage of data7.
- Conformity
Conformity measures how well data adheres to specific formatting, coding, and content guidelines.
- Completeness
The degree to which data captures all relevant information about a particular phenomenon or activity.
- Consistency
The degree to which data is free from contradictions or discrepancies with other data sources. Data architecture is critical for organizations because it helps ensure data consistency.
- Integrity
Data integrity is the property that data has not been retrieved or altered without authorization since creation and until disposal8.
E-records integrity service maintains information as entered/captured and is auditable (e.g., audit trails) to affirm its traceability.
- Validity
Validity is the extent to which data elements comply with internal or external standards, guidelines, or standard data definitions, including data type, size, format, and other features.
- Reliability
A record is reliable if its content can be trusted as a complete and accurate representation of the transaction, activities, or facts it attests to. It can be depended upon in the course of subsequent transactions and activities. (NARA)
Reliability refers to the quality and accuracy of the data being collected. Data collected can include incomplete, inaccurate, or unreliable data, so it's essential to ensure it is trustworthy before using it for analysis. Data validation9 is relevant to ensure the reliability of the collected data.
- Timely
Timely is the degree to which data is up-to-date and reflects the current state of the activity.
- Consistent
Consistent data ensures that the same information is represented in the same way, regardless of where it is used or accessed.
High-quality data is trustworthy10, relevant11, and fit for its intended purpose. Data quality can lead to correct insights, flawless decision-making, and decreased costs, risks, and efficiencies.
High-quality data requires proper management processes, including regular quality checks, cleaning, validation, and governance. Regulated companies should also invest in high-quality data management systems and training programs to ensure employees have the skills to manage data effectively.
Organizations can implement data quality frameworks, tools, and best practices to ensure that data is accurate, auditable, conform, complete, consistent, with integrity, valid, reliable, timely, and consistent. It meets the business and analytical needs of the organization.
Data integrity, data reliability, and data quality
Data with integrity, reliability and quality are essential for data management, but they refer to different data characteristics.
The worldwide regulatory agencies or competent authorities define data integrity as the “degree to which data are complete, consistent, accurate, trustworthy, reliable and that these characteristics of the data are maintained throughout the data life cycle.”
The key word in this definition is “trustworthy.” Trustworthy data is considered reliable, authentic, with integrity, and usable.
It is implied that reliable data is, as part of many attributes, with integrity. But integrity alone does not define reliable data. The definition of data integrity designated by worldwide regulatory agencies or competent authorities must be corrected and consistent with worldwide standards12.
Based on United States and worldwide standards such as the NIST SP 800-57P1, IEEE, ISO-17025, INFOSEC, 44 USC 3542, 36 CFR Part 1236, and others, data/e-records integrity is the property that data/e-records have not been retrieved or altered without authorization since created and until disposal. 21 CFR Part 211 requires that all raw data be recorded and maintained, even if the data is manipulated. The data integrity characteristic is accomplished by properly managing the raw data. Changes to the raw data must be traceable throughout the data life cycle, and security controls must be established for the computer system to ensure data protection. Any changes to the data should be documented as part of the metadata (e.g., audit trail).
To consider that data has integrity, the data must be protected from unauthorized changes, tampering, or corruption. Controls should be in place to ensure that data remains reliable, even when it is stored, moved, or integrated with other data sources.
Data security protects data from unauthorized access, use, or disclosure. It involves safeguarding data against unauthorized access or theft, ensuring data is available when needed, and protecting data from damage or loss.
Data is reliable if its content can be trusted as a complete and accurate representation of the transaction, activities, or facts it attests to. It can be depended upon during subsequent transactions and activities13.
Data reliability refers to the consistency and repeatability of data over time, regardless of the methods or tools used to collect it. A dataset is reliable if it produces consistent results, even if measured multiple times or obtained by different researchers or methods. For instance, if an experiment yields the same results every time it's performed, it is considered reliable.
Trusted is a term pertinent to reliability. Reliable data is accurate, authentic14, with integrity, usable, and can be confidently used for decision-making, analysis, and other purposes.
Trusted data is critical for making informed decisions and achieving positive outcomes. It requires a combination of data quality, integrity, and security to ensure that data is reliable, accurate, and trustworthy. Decisions based on reliable or accurate data can lead to good outcomes and negative consequences.
Several factors must be considered to ensure that data is trusted, including data quality, integrity, and security.
Data quality is a measure of how well data meets the requirements and expectations of users for their intended purpose.
Data quality is determined by several attributes, including accurate, auditable, conform, complete, consistent, with integrity, validity, reliable, timely, and consistent with the intended use. These attributes ensure making the data is trustworthy.
The data must be accurate and error-free, such as duplicates or missing values. The data must also be complete, with all required fields populated, consistent across all sources, and relevant to the problem being addressed.
High-quality data is free from errors, bias, and inconsistencies and relevant to the questions or decisions made. For instance, if a company's customer data is accurate, complete, and current, it can be used to make informed business decisions.
In summary, data reliability is focused on the consistency and repeatability of data, while data quality is concerned with the accuracy, completeness, and relevance of data to its intended use.
In the data set containing quality data, all the data set is also reliable. In a data set containing reliable data, the data set is also with integrity. But, in a data set containing data with integrity, only some of the data set is reliable. In a data set containing reliable data, only some of the data set is data with quality.
Comparison of Data Quality, ALCOA and others
The table depicts the dimensions of ALCOA, ALCOA+. ALCOA++, reliability, and Data Quality. Data Quality dimensions based on the EU medicinal regulations17 are listed for reference only.
The table also associates ALCOA with the attributes to assess the collected data quality and demonstrate the safety and efficacy of medicinal products.
From the table, it can be established that data requires at least four pillars to work:
- 1. Complete refers to data containing all the information required to make accurate and reliable conclusions.
- 2. Validity refers to whether the data accurately reflects what it claims to represent.
- 3. Timeliness refers to when the data was collected and when it is being used or analyzed.
- 4. Accurate refers to whether the data values stored for an object are correct. The accuracy characteristics are precise, truthful, and reflect what occurred.
It can be established the following relationships:
- 1. Data accuracy, precision, and reflection of what occurred contemporaneously are the outcome of data completeness. By adding "complete" to ALCOA, ALCOA+ is directly establishing this relationship.
- 2. If the data reflects what occurred during the data lifecycle, the data can be considered with integrity. The data has been protected from unauthorized changes, tampering, or corruption, even when stored, moved, or integrated with other data sources.<
- 3. The only two frameworks containing the dimension of traceability are ALCOA++ and Quality Data. It is relevant to the raw data18 changes that must be traceable throughout the data life cycle.
The controls related to data integrity can be summarized as follows:
- Security-related controls.
- Traceability of the modification to the raw data.
The controls related to data reliability can be summarized as follows:
- Data integrity controls
- Accurate
- Complete
- Consistent
Implementing workflows meeting 21 CFR Part 11.10(f) and data flows meeting the Annex 11-5 to the EU GMP guideline verifying the data accuracy, completeness, and consistency determine if data is missing or unusable.
The controls related to data quality can be summarized as follows:
- Data reliability-related controls.
- Consistency-related controls determine the lack of conflict with other data values and adherence to a standard format (e.g., conformity).
- Duplication-related controls determine the repeated records.
Based on the above, it is easy to determine that “the controls required for integrity do not necessarily guarantee the quality of the data generated”19.
The dimensions of ALCOA and ALCOA+ can be correlated to the data’s reliability dimensions. The dimensions related to ALCOA++ can be correlated to the dimensions of the quality of data.
Summary
The Data Quality attributes are not equivalent to the DI attributes. The “controls required for integrity do not necessarily guarantee the quality of the data generated”19.
The dimensions of ALCOA and ALCOA+ can be correlated to the data’s reliability dimensions. The dimensions related to ALCOA++ can be correlated to the dimensions of the quality of data.
References:
1 Attribute – any detail that serves to qualify, identify, quantify, or express the state of a relation or an entity.
2 https://www.linkedin.com/feed/update/urn:li:activity:7046255838855856128?commentUrn=urn%3Ali%3Acomment%3A%28activity%3A7046255838855856128%2C7047292986165968896%29
3 Data quality is defined as fitness for purpose for users’ needs about health research, policy-making, and regulation and that the data reflect the reality they aim to represent. (European Health Data Space Data Quality Framework, Deliverable 6.1 of TEHDAS EU 3rd Health 567 Program (GA: 101035467). May 18th, 2022)
4 EMA, “EMA Questions and answers: Good manufacturing practice Data Integrity,” Aug 2016.
5 López, O., “Introduction to Data Quality,” Journal of Validation Technology, April 2020.
6 Moses, B., Gavish, L., Vorwerck, M., “Data Quality Fundamentals - A Practitioner’s Guide to Building Trustworthy Data Pipelines”, O’Reilly Media, Inc., Sebastopol, CA, September 2022.
7 Data lineage represents information about everything that has “happened” to the data. Whether it was moved from one system to another, transformed, aggregated, etc., ETL (extraction, transformation, and load) tools can capture this metadata electronically. (DataManagementU)
8 NIST SP 800-57P1, IEEE, ISO-17025, INFOSEC, 44 USC 3542, 36 CFR Part 1236, and others standards.
9 Data Validation is the procedural control of verifying collected data/e-records by a second operator or by validated electronic means to ensure that data/e-records are consistent, accurate, and trustworthy. In addition, it is ensured that these data/e-records are accurately transcribed into machine-readable form.
10 Trustworthy data - Reliability, authenticity, integrity, and usability are the characteristics used to describe reliable data from a record management perspective. (NARA)
11 Data relevancy - Data relevance refers to the degree to which data is valuable, meaningful, and aligned with the business or analytical objectives.
12 Worldwide standards are a set of globally recognized specifications, guidelines, or requirements that define how products, services, or systems should be designed, manufactured, and operated to meet quality, safety, and performance criteria. International organizations develop these standards.
13 United States National Archives and Records Administration (https://www.archives.gov/)
14 Authenticity - The property of being genuine and being able to be verified and trusted; confidence in the validity of a transmission, a message, or message originator. See authentication. (NIST Special Publication 800-18)
15 A dimension represents one or more related aspects or features of reality.
16 A framework is a natural or conceptual structure intended to serve as a support or guide for the building of something that expands the structure into something useful
17 EMA, “Data quality framework EU medicines regulation,” September 2022 (draft).
18 Raw data - The original records (data) and certified true copies of original records, including source data and metadata and all subsequent transformations and reports of this data, which are recorded at the time of the GxP activity and allow complete reconstruction and evaluation of the GxP activity. (WHO)
19 MHRA, “GxP Data Integrity Guidance and Definitions,” March 2018.
About the Author
Orlando López...
is a seasoned expert with worldwide experience in pharmaceutical device and medical device e-compliance.